Edge AI technology runs artificial intelligence (AI) algorithms on local devices with edge computing capabilities that enable performing computation and data storage on user devices such as laptops, Internet of Things (IoT) devices, or dedicated edge servers. Unlike cloud environments, edge computing is not affected by bandwidth and latency issues.
Edge AI combines edge computing and AI to enable processing user data in real time. Without requiring systems to connect to other resources in the cloud or on-premises. Computation is done locally while the data remains on the device, ensuring the user’s privacy. So, users can curate their data first before it is sent to a different location.
How Edge AI Works
In the not-so-distant past, AI applications were often developed using symbolic AI techniques. That hard-code rules into applications such as fraud detection algorithms and expert systems. Nonsymbolic AI techniques like neural networks were developed for use cases. Like optical character recognition (OCR) for typed text or check numbers.
The next evolution in AI was the discovery of inferencing. Which enables scaling up deep neural networks (DNN) in the cloud to train AI models. So, generate responses according to input data. Edge AI takes AI development further by enabling deployment outside cloud environments. Today, data scientists use cloud AI to train new algorithms and edge AI for inferencing.
Inferencing algorithms require less processing capabilities and computing power compared to the resources needed for training algorithms. Well-designed inferencing algorithms can run on existing CPUs or less capable microcontrollers in edge devices. There are also highly efficient AI chips that can reduce power and improve inferencing performance or achieve both goals.
The importance of model monitoring for Edge AI
Model monitoring is an essential aspect of Edge AI. As it involves the monitoring and management of machine learning models deployed at the edge. This includes tracking the performance of the model, detecting and diagnosing issues, and making updates or adjustments as needed.
ML model monitoring in edge AI includes a variety of tasks. Such as checking the accuracy and performance of the model, identifying and diagnosing issues. Updating the model’s parameters and weights.
What Are the Benefits of Edge AI?
AI algorithms can understand various forms of unstructured information. Including languages, sounds, sights, smells, faces, and temperature, making them especially useful for end users. Here are several examples:
- Intelligence: Unlike conventional applications that can respond solely to the inputs anticipated. By the programmer, AI applications can function more flexibly and adaptively. For example, an AI neural network is trained to answer a certain type of question rather than a specific question. Ensuring it can reply even if the question is new.
- Real-time insights: Edge technology can analyze data locally instead of doing so remotely in a cloud environment. By eliminating the delays typically caused due to long-distance communications, edge technology can respond to users’ needs in real-time.
- Reduced cost: Edge technology brings processing power to the edge, minimizing demands for Internet bandwidth and significantly reducing networking costs.
- Increased privacy: Performing AI at the edge enables analyzing real-world information. Without exposing it to human operators, significantly increasing the privacy of users. Whose personal information, such as appearance, medical image, or voice, is analyzed. Edge AI improves data privacy by keeping it locally and uploading only the analysis and insights to the cloud. Further simplifying regulatory data compliance.
- High availability: Edge AI is up with decentralization and offline capabilities. That eliminate the need for Internet access for data processing. It supports mission-critical, production-grade AI applications with higher availability and reliability.
- Persistent improvement: AI models can become more accurate by training on additional data. An edge AI application that confronts data it cannot accurately or confidently process typically uploads the data for retraining. As a result, the longer an AI model is in production at the edge, the more accurate it can become.
What are the Challenges of Edge AI?
Edge AI can present several challenges, including:
- Limited computational power: Edge devices and IoT devices often have limited computational power and memory, which can make it challenging to run complex AI algorithms.
- Limited storage capacity: Many edge devices have limited storage capacity, making it challenging to store large amounts of data for training AI models.
- Limited connectivity: Edge devices may not have a constant or reliable internet connection, making it challenging to update AI models or send data to a central location for processing.
- Power constraints: Edge devices may have limited power resources, making it challenging to run AI algorithms that require significant computational power.
- Data privacy and security: Edge AI systems can generate and process sensitive data, making it important to ensure that data is properly protected and that systems comply with relevant regulations.
- Integration and maintenance: Integrating Edge AI systems with existing systems can be complex and time-consuming, and maintaining Edge AI systems can be challenging due to the distributed nature of the systems.
- Lack of standardization: Edge AI is still a relatively new field, and there is currently a lack of standardization in terms of the technologies and protocols used.
- Difficulty in finding expert talent: Edge AI is a niche field and it may be difficult to find personnel with the necessary skills and expertise to design and maintain Edge AI systems.
Despite these challenges, Edge AI is a rapidly growing field, and new technologies and techniques are constantly being under development to address these challenges and make it easier to implement Edge AI systems.
Conclusion
In conclusion, edge AI enables data scientists to run AI algorithms at the edge. It ensures the privacy and security of user data by performing analysis on local devices, and uploading only insights to cloud environments. This analysis can be up in real-time because everything is up locally and there is no need to communicate with distant cloud environments.
Organizations can leverage edge AI to gain access to insights while maintaining data privacy and reducing the costs associated with networking costs. However, as the technology is relatively new, organizations might find it difficult to find expert talent, and data scientists might encounter challenges due to the lack of standardization.
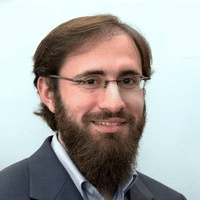
Author Bio: Gilad David Maayan
Gilad David Maayan is a technology writer who has worked with over 150 technology companies including SAP, Imperva, Samsung NEXT, NetApp and Check Point, producing technical and thought leadership content that elucidates technical solutions for developers and IT leadership. Today he heads Agile SEO, the leading marketing agency in the technology industry.
The post What Is Edge AI? appeared first on Visualmodo.
0 Commentaires